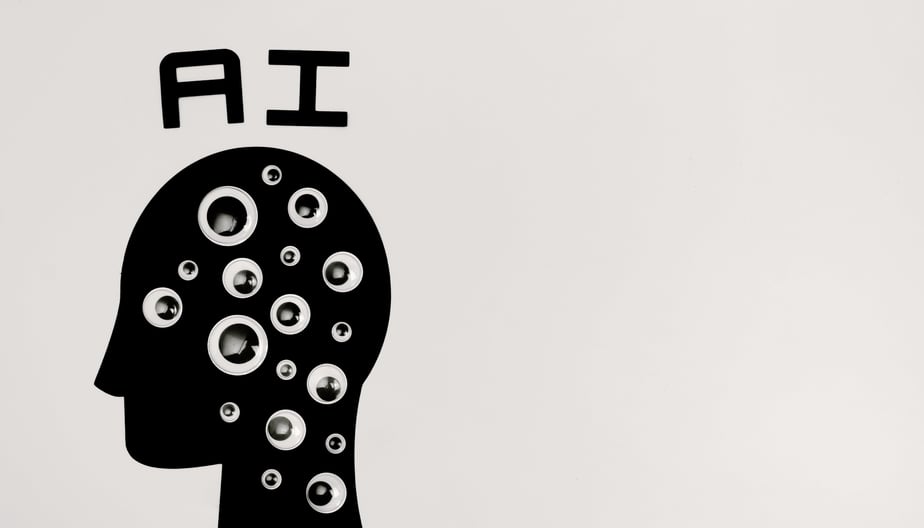
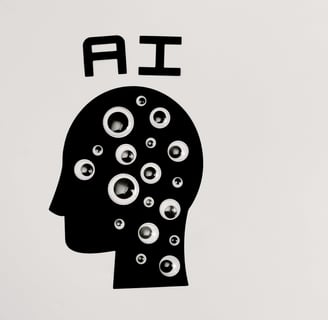
Introduction
In the rapidly advancing realm of artificial intelligence (AI), the intersection of ethics, fairness, and bias mitigation has emerged as a pivotal area of research. As AI systems become integral to diverse sectors of society, the imperative to ensure their ethical behavior and fairness becomes paramount. This essay embarks on a comprehensive exploration of the key aspects of this research, delving into ethical considerations, strategies for fairness and bias mitigation, and the challenges inherent in achieving these goals. From healthcare to finance, criminal justice to employment, the impact of ethical AI reverberates across sectors, influencing decision-making processes and societal outcomes.
Ethical AI
At the heart of ethical AI lies a commitment to imbue artificial intelligence systems with principles that align with human values. This involves not only considering the technical aspects of AI development but also the broader societal implications of its deployment. Ethical AI research seeks to establish guidelines and frameworks that guide developers and policymakers in creating AI systems that prioritize fairness, transparency, and accountability. The goal is to ensure that AI technologies contribute positively to society, respecting fundamental human rights and avoiding harm to individuals or communities. By embedding ethical considerations into the fabric of AI development, researchers aspire to navigate the complex ethical landscape and build technology that is a force for good.
However, achieving ethical AI is not without its challenges. The dynamic and evolving nature of AI technologies presents a moving target for ethical standards. Researchers grapple with questions of how to balance competing ethical principles, navigate cultural nuances, and ensure that AI systems are aligned with diverse perspectives. The ethical considerations extend beyond the development phase to encompass the entire lifecycle of AI, including deployment, monitoring, and adaptation. Striking a balance between innovation and ethical responsibility is a delicate task, demanding ongoing dialogue and collaboration between technologists, ethicists, policymakers, and the broader society.
Fairness and Bias Mitigation
Fairness in AI is a cornerstone in the quest for ethical deployment. Machine learning models trained on biased data or with inherent biases in their algorithms can perpetuate and amplify societal inequalities. Researchers, therefore, focus on developing methodologies to identify, quantify, and mitigate biases in AI systems. Algorithmic fairness becomes a central concern, demanding the creation of models that provide equitable outcomes across diverse demographic groups. Achieving fairness is a multifaceted challenge, as biases can originate from various sources, including biased training data, algorithmic design choices, or inherent societal prejudices.
Addressing data bias is a critical aspect of fairness in AI. Training datasets often mirror existing societal biases, leading to skewed model predictions. Researchers are actively developing techniques to preprocess training data, identify biased patterns, and introduce mechanisms that correct for these imbalances. Moreover, algorithmic fairness involves creating models that are not only accurate but also exhibit fairness in their predictions across different subgroups. Striking a balance between accuracy and fairness is a nuanced task, requiring careful consideration of trade-offs and the impact on different demographic groups.
Transparency and explainability play pivotal roles in the fairness equation. Understanding how AI systems arrive at decisions is essential for detecting and rectifying biases. Researchers are exploring ways to make AI models more interpretable, enabling users to comprehend the decision-making process and identify potential biases. Explainability also contributes to building trust in AI technologies, as users, stakeholders, and affected communities can better understand and scrutinize the functioning of these systems. The journey towards fairness in AI involves a continuous refinement of methodologies, models, and evaluation metrics to ensure that AI systems contribute to equitable outcomes.
Challenges and Considerations
The pursuit of ethical AI, fairness, and bias mitigation is not without its challenges. One of the primary obstacles is the pervasive nature of biases in training data. Researchers are confronted with the task of identifying and rectifying biases deeply ingrained in datasets, a process that requires meticulous attention to detail and an understanding of the societal contexts that contribute to these biases. Additionally, the challenge extends to striking a balance between fairness and model performance. Ensuring fair outcomes without compromising the accuracy and effectiveness of AI systems demands a nuanced approach that considers the specific requirements of different applications.
Another significant challenge lies in the interpretability and explainability of AI models. As AI systems become more complex, understanding the decision-making processes becomes increasingly challenging. Researchers are actively exploring methods to enhance the interpretability of models, making them more accessible to users and stakeholders who may not have a technical background. Balancing the need for sophisticated, high-performing models with the imperative for transparency is an ongoing consideration in the realm of ethical AI.
The ethical considerations extend beyond technical challenges to encompass societal and cultural dimensions. Different communities may have diverse perspectives on what constitutes fairness, and incorporating these perspectives into AI development is crucial for avoiding biased outcomes. Cultural competency and inclusivity become key aspects of ethical AI research, emphasizing the importance of diverse representation and collaboration with affected communities in the design and evaluation of AI systems.
Applications
The impact of ethical AI, fairness, and bias mitigation is palpable across various domains. In healthcare, ethical considerations shape the development of diagnostic and treatment recommendation systems. Fairness is essential to prevent healthcare disparities, ensuring that AI-driven applications contribute to equitable outcomes for patients regardless of their demographic background. The responsible deployment of AI in healthcare has the potential to enhance diagnosis, treatment planning, and patient care while minimizing disparities in access to quality healthcare.
Finance is another sector where the ethical use of AI is of paramount importance. Fairness considerations are critical in credit scoring, loan approval processes, and other financial decisions. Ethical AI research aims to eliminate biases that may arise from historical data, systemic inequalities, or discriminatory practices. By ensuring fairness, researchers seek to create financial systems that provide equal opportunities to individuals, irrespective of their background or demographic characteristics.
In the realm of criminal justice, ethical AI research plays a crucial role in preventing the amplification of biases in law enforcement and judicial decision-making. Fairness considerations are integral to avoid perpetuating existing disparities and ensuring that AI technologies contribute to just and equitable outcomes. Ethical AI applications in criminal justice extend to risk assessment tools, sentencing guidelines, and policing strategies, with the goal of fostering fairness, transparency, and accountability.
The employment and hiring landscape also witnesses the impact of ethical AI research. Bias mitigation is essential in recruitment algorithms to ensure that hiring decisions are based on merit, skills, and qualifications rather than perpetuating existing biases. Ethical considerations in AI for employment encompass promoting diversity and equal opportunities in the workforce, addressing historical disparities, and creating inclusive hiring practices.
Conclusion
In conclusion, the dynamic field of ethical AI, fairness, and bias mitigation in machine learning models represents a collective effort to address the ethical challenges associated with the deployment of AI technologies. As these technologies become integral to various aspects of society, it becomes imperative to ensure that they adhere to ethical principles, promote fairness, and mitigate biases. The journey towards ethical AI involves a multidimensional exploration, encompassing technical, societal, and cultural dimensions.
From embedding ethical considerations into the development process to actively mitigating biases in algorithms, researchers navigate a complex terrain that demands continual refinement and innovation. The challenges are significant, from addressing biases in training data to balancing fairness with model performance. However, the potential impact is transformative, shaping a future where AI technologies contribute positively to society, foster fairness, and align with human values.
As applications span across healthcare, finance, criminal justice, and employment, ethical AI research influences decision-making processes that impact individuals and communities. The ethical deployment of AI is not only a technical endeavor but also a societal responsibility. By engaging in ongoing dialogue, collaborating with diverse stakeholders, and embracing inclusivity, the research into ethical AI, fairness, and bias mitigation paves the way for a future where AI technologies are not only powerful but also just, equitable, and aligned with the principles of a fair and inclusive society.
Read also - https://www.admit360.in/equitable-healthcare-access-outcomes